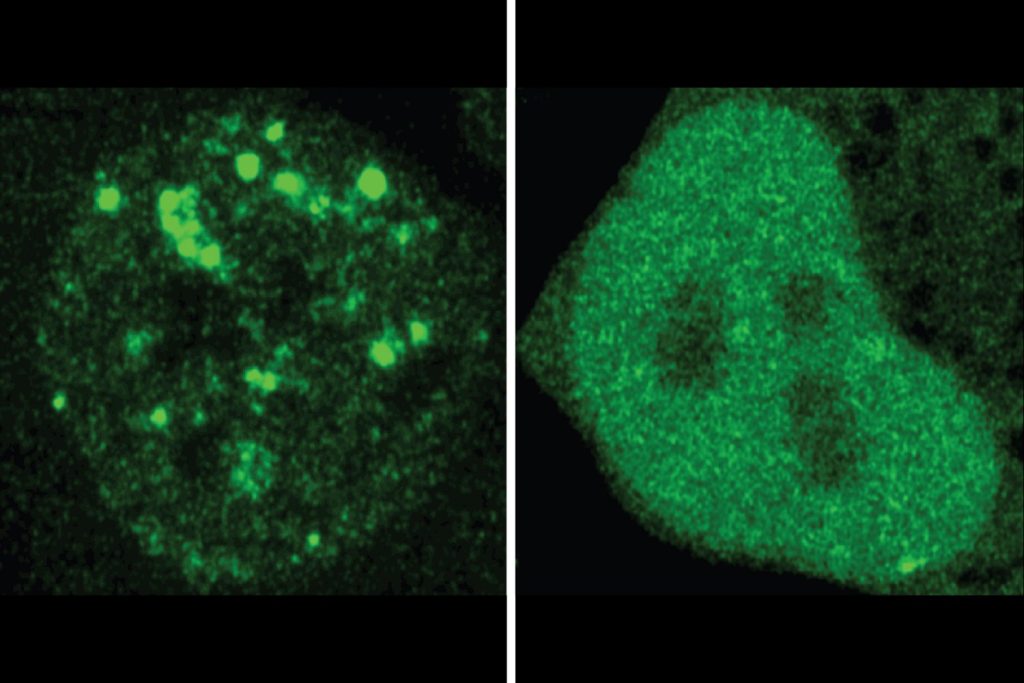
Proteins are the workhorses that hold our cells operating, and there are a lot of 1000’s of forms of proteins in our cells, every performing a specialised operate. Researchers have lengthy recognized that the construction of a protein determines what it will possibly do. Extra lately, researchers are coming to understand {that a} protein’s localization can also be vital for its operate. Cells are filled with compartments that assist to prepare their many denizens. Together with the well-known organelles that adorn the pages of biology textbooks, these areas additionally embody quite a lot of dynamic, membrane-less compartments that focus sure molecules collectively to carry out shared capabilities. Realizing the place a given protein localizes, and who it co-localizes with, can subsequently be helpful for higher understanding that protein and its function within the wholesome or diseased cell, however researchers have lacked a scientific option to predict this data.
In the meantime, protein construction has been studied for over half-a-century, culminating within the synthetic intelligence software AlphaFold, which may predict protein construction from a protein’s amino acid code, the linear string of constructing blocks inside it that folds to create its construction. AlphaFold and fashions prefer it have turn into broadly used instruments in analysis.
Proteins additionally include areas of amino acids that don’t fold into a set construction, however are as an alternative vital for serving to proteins be part of dynamic compartments within the cell. MIT Professor Richard Younger and colleagues puzzled whether or not the code in these areas might be used to foretell protein localization in the identical manner that different areas are used to foretell construction. Different researchers have found some protein sequences that code for protein localization, and a few have begun growing predictive fashions for protein localization. Nonetheless, researchers didn’t know whether or not a protein’s localization to any dynamic compartment might be predicted primarily based on its sequence, nor did they’ve a comparable software to AlphaFold for predicting localization.
Now, Younger, additionally member of the Whitehead Institute for Organic Analysis; Younger lab postdoc Henry Kilgore; Regina Barzilay, the College of Engineering Distinguished Professor for AI and Well being in MIT’s Division of Electrical Engineering and Pc Science and principal investigator within the Pc Science and Synthetic Intelligence Laboratory (CSAIL); and colleagues have constructed such a mannequin, which they name ProtGPS. In a paper printed on Feb. 6 within the journal Science, with first authors Kilgore and Barzilay lab graduate college students Itamar Chinn, Peter Mikhael, and Ilan Mitnikov, the cross-disciplinary crew debuts their mannequin. The researchers present that ProtGPS can predict to which of 12 recognized forms of compartments a protein will localize, in addition to whether or not a disease-associated mutation will change that localization. Moreover, the analysis crew developed a generative algorithm that may design novel proteins to localize to particular compartments.
“My hope is that it is a first step in direction of a robust platform that allows folks finding out proteins to do their analysis,” Younger says, “and that it helps us perceive how people grow to be the advanced organisms that they’re, how mutations disrupt these pure processes, and how one can generate therapeutic hypotheses and design medicine to deal with dysfunction in a cell.”
The researchers additionally validated most of the mannequin’s predictions with experimental exams in cells.
“It actually excited me to have the ability to go from computational design all the best way to making an attempt these items within the lab,” Barzilay says. “There are numerous thrilling papers on this space of AI, however 99.9 % of these by no means get examined in actual techniques. Due to our collaboration with the Younger lab, we had been in a position to check, and actually learn the way effectively our algorithm is doing.”
Creating the mannequin
The researchers skilled and examined ProtGPS on two batches of proteins with recognized localizations. They discovered that it might accurately predict the place proteins find yourself with excessive accuracy. The researchers additionally examined how effectively ProtGPS might predict adjustments in protein localization primarily based on disease-associated mutations inside a protein. Many mutations — adjustments to the sequence for a gene and its corresponding protein — have been discovered to contribute to or trigger illness primarily based on affiliation research, however the methods through which the mutations result in illness signs stay unknown.
Determining the mechanism for the way a mutation contributes to illness is vital as a result of then researchers can develop therapies to repair that mechanism, stopping or treating the illness. Younger and colleagues suspected that many disease-associated mutations may contribute to illness by altering protein localization. For instance, a mutation might make a protein unable to affix a compartment containing important companions.
They examined this speculation by feeding ProtGOS greater than 200,000 proteins with disease-associated mutations, after which asking it to each predict the place these mutated proteins would localize and measure how a lot its prediction modified for a given protein from the conventional to the mutated model. A big shift within the prediction signifies a probable change in localization.
The researchers discovered many circumstances through which a disease-associated mutation appeared to vary a protein’s localization. They examined 20 examples in cells, utilizing fluorescence to match the place within the cell a traditional protein and the mutated model of it ended up. The experiments confirmed ProtGPS’s predictions. Altogether, the findings help the researchers’ suspicion that mis-localization could also be an underappreciated mechanism of illness, and reveal the worth of ProtGPS as a software for understanding illness and figuring out new therapeutic avenues.
“The cell is such a sophisticated system, with so many elements and sophisticated networks of interactions,” Mitnikov says. “It’s tremendous fascinating to assume that with this strategy, we are able to perturb the system, see the result of that, and so drive discovery of mechanisms within the cell, and even develop therapeutics primarily based on that.”
The researchers hope that others start utilizing ProtGPS in the identical manner that they use predictive structural fashions like AlphaFold, advancing numerous initiatives on protein operate, dysfunction, and illness.
Transferring past prediction to novel era
The researchers had been excited concerning the doable makes use of of their prediction mannequin, however additionally they wished their mannequin to transcend predicting localizations of current proteins, and permit them to design fully new proteins. The aim was for the mannequin to make up totally new amino acid sequences that, when fashioned in a cell, would localize to a desired location. Producing a novel protein that may really accomplish a operate — on this case, the operate of localizing to a selected mobile compartment — is extremely tough. In an effort to enhance their mannequin’s possibilities of success, the researchers constrained their algorithm to solely design proteins like these present in nature. That is an strategy generally utilized in drug design, for logical causes; nature has had billions of years to determine which protein sequences work effectively and which don’t.
Due to the collaboration with the Younger lab, the machine studying crew was in a position to check whether or not their protein generator labored. The mannequin had good outcomes. In a single spherical, it generated 10 proteins meant to localize to the nucleolus. When the researchers examined these proteins within the cell, they discovered that 4 of them strongly localized to the nucleolus, and others might have had slight biases towards that location as effectively.
“The collaboration between our labs has been so generative for all of us,” Mikhael says. “We’ve discovered how one can communicate one another’s languages, in our case discovered so much about how cells work, and by having the possibility to experimentally check our mannequin, we’ve been in a position to determine what we have to do to truly make the mannequin work, after which make it work higher.”
Having the ability to generate practical proteins on this manner might enhance researchers’ capability to develop therapies. For instance, if a drug should work together with a goal that localizes inside a sure compartment, then researchers might use this mannequin to design a drug to additionally localize there. This could make the drug simpler and reduce unintended effects, for the reason that drug will spend extra time participating with its goal and fewer time interacting with different molecules, inflicting off-target results.
The machine studying crew members are enthused concerning the prospect of utilizing what they’ve discovered from this collaboration to design novel proteins with different capabilities past localization, which might broaden the chances for therapeutic design and different functions.
“Quite a lot of papers present they’ll design a protein that may be expressed in a cell, however not that the protein has a specific operate,” Chinn says. “We really had practical protein design, and a comparatively large success charge in comparison with different generative fashions. That’s actually thrilling to us, and one thing we wish to construct on.”
The entire researchers concerned see ProtGPS as an thrilling starting. They anticipate that their software shall be used to be taught extra concerning the roles of localization in protein operate and mis-localization in illness. As well as, they’re fascinated by increasing the mannequin’s localization predictions to incorporate extra forms of compartments, testing extra therapeutic hypotheses, and designing more and more practical proteins for therapies or different functions.
“Now that we all know that this protein code for localization exists, and that machine studying fashions could make sense of that code and even create practical proteins utilizing its logic, that opens up the door for therefore many potential research and functions,” Kilgore says.