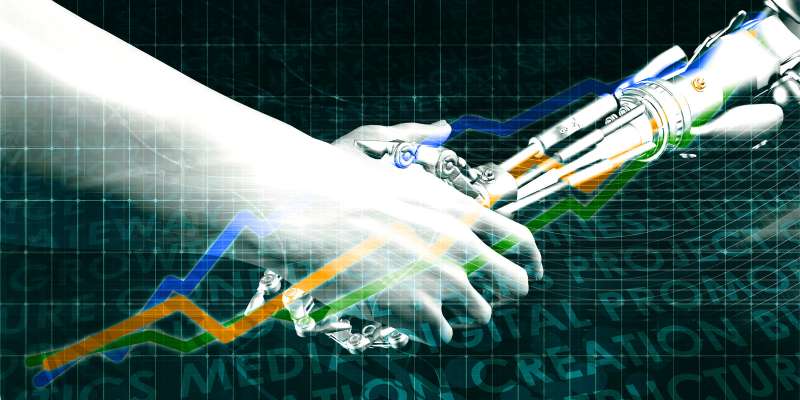
The Function of Machine Studying in Portfolio Optimization
Introduction:
The world of finance has lengthy been dominated by conventional funding methods, typically primarily based on inflexible algorithms and guide knowledge evaluation. Nonetheless, the appearance of machine studying (ML) has revolutionized the business, particularly in portfolio optimization. By combining huge quantities of knowledge with superior algorithms, machine studying affords the power to make smarter, quicker, and extra correct funding choices. On this article, I’ll discover how machine studying in portfolio optimization is reshaping the panorama of funding administration, its advantages, challenges, and real-world functions.
Understanding Portfolio Optimization
Earlier than diving into the position of machine studying, it’s important to know what portfolio optimization is. At its core, portfolio optimization goals to seek out the best steadiness between threat and return for an funding portfolio. The objective is to maximise returns whereas minimizing threat, typically utilizing mathematical fashions to realize this steadiness.
Conventional Portfolio Optimization
Historically, portfolio optimization has relied on fashions similar to Fashionable Portfolio Idea (MPT), which emphasizes diversification to cut back threat. The environment friendly frontier, an idea launched by Harry Markowitz, helps buyers steadiness threat and return by optimizing the allocation of property. Whereas these fashions have been instrumental in portfolio administration, they typically fall quick within the face of complicated market circumstances and quickly altering monetary environments.
The Want for Machine Studying
The limitation of conventional fashions is that they depend on static assumptions and human intervention. Machine studying affords an answer by enabling real-time knowledge processing and adaptive decision-making. It will possibly constantly study from new market knowledge and alter funding methods accordingly.
The Fundamentals of Machine Studying
To totally respect how machine studying enhances portfolio optimization, we should first perceive what machine studying is and the way it works.
What’s Machine Studying?
Machine studying is a subset of synthetic intelligence (AI) that focuses on constructing methods that may study from knowledge, enhance over time, and make predictions with out being explicitly programmed. It entails the usage of algorithms to research massive units of knowledge, determine patterns, and make choices primarily based on that evaluation.
Sorts of Machine Studying
There are three major forms of machine studying:
- Supervised Studying: The mannequin is educated utilizing labeled knowledge and learns to foretell outcomes primarily based on that knowledge.
- Unsupervised Studying: The mannequin identifies hidden patterns in knowledge with none prior labels.
- Reinforcement Studying: The mannequin learns by interacting with the atmosphere and receiving suggestions primarily based on its actions.
Why Machine Studying is Essential in Finance
In finance, machine studying permits for extra correct forecasting, more practical threat administration, and a greater understanding of market tendencies. The power to course of large quantities of knowledge in actual time offers buyers a aggressive edge and helps optimize portfolios with precision.
Purposes of Machine Studying in Portfolio Optimization
Machine studying is already making waves in portfolio optimization, bringing a wealth of advantages to asset managers and buyers alike. Right here’s how ML is utilized:
Threat Evaluation and Administration: One of the vital highly effective functions of machine studying is in threat administration. Conventional threat fashions are sometimes primarily based on historic knowledge and static assumptions. In distinction, machine studying can course of huge quantities of real-time knowledge and predict potential dangers with a lot larger accuracy. This allows portfolio managers to anticipate market shifts and make changes earlier than dangers materialize.
For instance, ML algorithms can analyze patterns in monetary markets to forecast volatility and alter a portfolio’s publicity to totally different asset courses accordingly.
Asset Allocation: Machine studying is used to reinforce asset allocation methods. By analyzing historic knowledge, financial indicators, and real-time market info, ML fashions can advocate optimum allocations for various asset varieties—equities, bonds, commodities, and extra.
The algorithms constantly adapt to altering market circumstances, guaranteeing that the portfolio stays aligned with the investor’s threat tolerance and goals.
Predictive Analytics for Returns: Machine studying can be used to foretell inventory returns and market tendencies. By analyzing historic inventory costs, financial knowledge, and monetary indicators, ML algorithms can determine patterns and correlations that conventional fashions might overlook. This predictive functionality permits for extra knowledgeable decision-making when deciding on property for a portfolio.
Furthermore, NLP in monetary information permits machine studying algorithms to research unstructured knowledge, similar to information articles, earnings reviews, and market sentiment, additional bettering the accuracy of predictions.
Rebalancing Portfolios: Portfolio rebalancing entails adjusting the composition of property to keep up a desired stage of threat and return. ML algorithms assist automate this course of by constantly monitoring market circumstances and portfolio efficiency, making rebalancing choices in actual time primarily based on pre-defined guidelines or objectives.
Portfolio Customization: Machine studying additionally permits personalized portfolios tailor-made to particular person buyers. By analyzing an investor’s preferences, threat tolerance, and monetary objectives, ML fashions can create portfolios which are aligned with their distinctive necessities.
Advantages of Machine Studying in Portfolio Optimization
Machine studying’s affect on portfolio optimization is profound, providing a number of advantages that improve each efficiency and effectivity:
Improved Determination-Making: Machine studying can course of massive datasets rapidly and determine patterns that may take a human analyst years to uncover. This results in extra knowledgeable and correct funding choices.
Dealing with Massive Datasets: Monetary markets generate large quantities of knowledge each second. Machine studying can effectively course of and analyze this knowledge, making it doable for portfolio managers to make choices primarily based on real-time info slightly than counting on outdated knowledge.
Actual-Time Evaluation: ML fashions can present real-time evaluation, which is essential for staying forward of market fluctuations. This allows buyers to answer modifications rapidly and alter their portfolios accordingly.
Higher Threat-Return Tradeoff: Machine studying’s capacity to dynamically alter portfolio allocations primarily based on altering circumstances ensures a greater risk-return tradeoff. This may end up in portfolios that obtain increased returns with out taking up extreme threat.
Challenges and Limitations of Machine Studying in Portfolio Optimization
Regardless of its many advantages, machine studying in portfolio optimization shouldn’t be with out its challenges:
Knowledge High quality and Availability: Machine studying algorithms rely closely on high-quality knowledge. The supply of fresh, related knowledge is crucial for the accuracy of predictions. Inaccurate or incomplete knowledge can result in poor decision-making and losses.
Overfitting and Mannequin Accuracy: One of many dangers of machine studying fashions is overfitting, the place a mannequin is just too intently aligned with historic knowledge, making it much less efficient in predicting future tendencies. It is a crucial subject in portfolio optimization, as market circumstances can change quickly.
Complexity of Algorithms: The complexity of machine studying fashions requires specialised information to implement and interpret. Whereas the know-how has made vital advances, the necessity for expert professionals to handle these fashions remains to be excessive.
Market Uncertainty: Machine studying fashions are constructed on historic knowledge, and whereas they’re glorious at predicting patterns primarily based on the previous, they might wrestle to adapt to sudden, unexpected market modifications or crises.
Actual-World Examples of Machine Studying in Portfolio Optimization
Machine studying has already discovered sensible functions within the funding world:
Hedge Funds and Institutional Buyers: Many hedge funds and institutional buyers have adopted machine studying fashions to optimize their portfolios. For instance, corporations like Two Sigma and Renaissance Applied sciences use ML algorithms to handle billions of {dollars} in property.
Retail Buyers and Robo-Advisors: Retail buyers profit from robo-advisors powered by machine studying. These platforms, similar to Betterment and Wealthfront, use algorithms to create and handle customized portfolios with little human intervention.
Progressive ML Fashions: A number of revolutionary ML fashions are getting used for portfolio optimization, similar to reinforcement studying algorithms that constantly adapt and study from new knowledge.
The Way forward for Machine Studying in Portfolio Optimization
The way forward for machine studying in portfolio optimization is vibrant. We will count on developments in AI applied sciences, together with higher predictive fashions, integration with huge knowledge, and real-time adaptation to altering market circumstances. Profitable AI funding methods will turn into extra exact, making it doable for buyers to realize their monetary objectives with larger effectivity.
Tendencies and Improvements: Anticipate the rise of AI in threat administration instruments that may combine extra superior knowledge sources, together with real-time financial indicators and international information feeds. These improvements will present buyers with even larger insights into their portfolios and the market.
Integration with Different Applied sciences: The longer term will see additional integration of machine studying with applied sciences similar to blockchain and quantum computing. These developments will assist optimize portfolios much more effectively, enabling a stage of precision that we can’t but totally predict.
Conclusion
Machine studying is essentially altering the panorama of portfolio optimization. From predictive analytics for returns to extra environment friendly threat administration, machine studying is driving smarter funding choices. Whereas challenges stay, the potential advantages—quicker, extra correct predictions, and higher risk-adjusted returns—are immense. As machine studying continues to evolve, its position in funding administration will solely develop, providing buyers new alternatives for fulfillment.