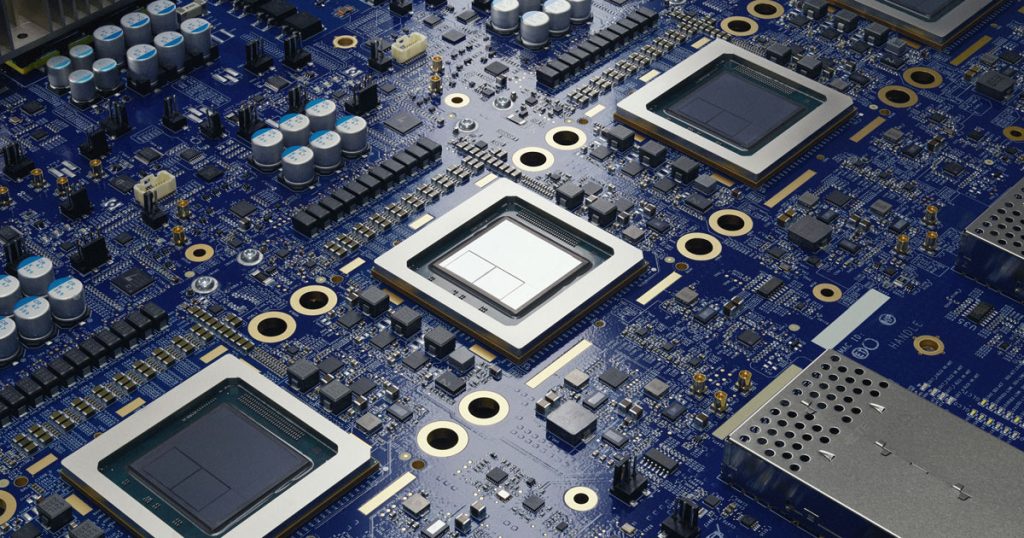
Analysis
Our AI technique has accelerated and optimized chip design, and its superhuman chip layouts are utilized in {hardware} around the globe
In 2020, we launched a preprint introducing our novel reinforcement studying technique for designing chip layouts, which we later revealed in Nature and open sourced.
As we speak, we’re publishing a Nature addendum that describes extra about our technique and its affect on the sphere of chip design. We’re additionally releasing a pre-trained checkpoint, sharing the mannequin weights and asserting its identify: AlphaChip.
Laptop chips have fueled exceptional progress in synthetic intelligence (AI), and AlphaChip returns the favor by utilizing AI to speed up and optimize chip design. The tactic has been used to design superhuman chip layouts within the final three generations of Google’s customized AI accelerator, the Tensor Processing Unit (TPU).
AlphaChip was one of many first reinforcement studying approaches used to resolve a real-world engineering downside. It generates superhuman or comparable chip layouts in hours, slightly than taking weeks or months of human effort, and its layouts are utilized in chips everywhere in the world, from information facilities to cell phones.
“
AlphaChip’s groundbreaking AI strategy revolutionizes a key section of chip design.
SR Tsai, Senior Vice President of MediaTek
How AlphaChip works
Designing a chip structure isn’t a easy process. Laptop chips include many interconnected blocks, with layers of circuit parts, all related by extremely skinny wires. There are additionally numerous complicated and intertwined design constraints that each one should be met on the identical time. Due to its sheer complexity, chip designers have struggled to automate the chip floorplanning course of for over sixty years.
Much like AlphaGo and AlphaZero, which discovered to grasp the video games of Go, chess and shogi, we constructed AlphaChip to strategy chip floorplanning as a sort of recreation.
Ranging from a clean grid, AlphaChip locations one circuit element at a time till it’s finished putting all of the parts. Then it’s rewarded primarily based on the standard of the ultimate structure. A novel “edge-based” graph neural community permits AlphaChip to be taught the relationships between interconnected chip parts and to generalize throughout chips, letting AlphaChip enhance with every structure it designs.
Left: Animation exhibiting AlphaChip putting the open-source, Ariane RISC-V CPU, with no prior expertise. Proper: Animation exhibiting AlphaChip putting the identical block after having practiced on 20 TPU-related designs.
Utilizing AI to design Google’s AI accelerator chips
AlphaChip has generated superhuman chip layouts utilized in each era of Google’s TPU since its publication in 2020. These chips make it doable to massively scale-up AI fashions primarily based on Google’s Transformer structure.
TPUs lie on the coronary heart of our highly effective generative AI techniques, from massive language fashions, like Gemini, to picture and video turbines, Imagen and Veo. These AI accelerators additionally lie on the coronary heart of Google’s AI companies and are obtainable to exterior customers through Google Cloud.
A row of Cloud TPU v5p AI accelerator supercomputers in a Google information middle.
To design TPU layouts, AlphaChip first practices on a various vary of chip blocks from earlier generations, corresponding to on-chip and inter-chip community blocks, reminiscence controllers, and information transport buffers. This course of known as pre-training. Then we run AlphaChip on present TPU blocks to generate high-quality layouts. Not like prior approaches, AlphaChip turns into higher and sooner because it solves extra situations of the chip placement process, just like how human specialists do.
With every new era of TPU, together with our newest Trillium (sixth era), AlphaChip has designed higher chip layouts and offered extra of the general floorplan, accelerating the design cycle and yielding higher-performance chips.
Bar graph exhibiting the variety of AlphaChip designed chip blocks throughout three generations of Google’s Tensor Processing Models (TPU), together with v5e, v5p and Trillium.
Bar graph exhibiting AlphaChip’s common wirelength discount throughout three generations of Google’s Tensor Processing Models (TPUs), in comparison with placements generated by the TPU bodily design staff.
AlphaChip’s broader affect
AlphaChip’s affect might be seen by means of its purposes throughout Alphabet, the analysis neighborhood and the chip design business. Past designing specialised AI accelerators like TPUs, AlphaChip has generated layouts for different chips throughout Alphabet, corresponding to Google Axion Processors, our first Arm-based general-purpose information middle CPUs.
Exterior organizations are additionally adopting and constructing on AlphaChip. For instance, MediaTek, one of many prime chip design corporations on the planet, prolonged AlphaChip to speed up growth of their most superior chips whereas enhancing energy, efficiency and chip space.
AlphaChip has triggered an explosion of labor on AI for chip design, and has been prolonged to different crucial levels of chip design, corresponding to logic synthesis and macro choice.
“
AlphaChip has impressed a completely new line of analysis on reinforcement studying for chip design, chopping throughout the design circulate from logic synthesis to floorplanning, timing optimization and past.
Professor Siddharth Garg, NYU Tandon College of Engineering
Creating the chips of the long run
We imagine AlphaChip has the potential to optimize each stage of the chip design cycle, from pc structure to manufacturing — and to rework chip design for customized {hardware} present in on a regular basis gadgets corresponding to smartphones, medical gear, agricultural sensors and extra.
Future variations of AlphaChip at the moment are in growth and we look ahead to working with the neighborhood to proceed revolutionizing this space and produce a few future during which chips are even sooner, cheaper and extra power-efficient.
Acknowledgements
We’re so grateful to our wonderful coauthors: Mustafa Yazgan, Joe Wenjie Jiang, Ebrahim Songhori, Shen Wang, Younger-Joon Lee, Eric Johnson, Omkar Pathak, Azade Nazi, Jiwoo Pak, Andy Tong, Kavya Srinivasa, William Grasp, Emre Tuncer, Quoc V. Le, James Laudon, Richard Ho, Roger Carpenter and Jeff Dean.
We particularly recognize Joe Wenjie Jiang, Ebrahim Songhori, Younger-Joon Lee, Roger Carpenter, and Sergio Guadarrama’s continued efforts to land this manufacturing affect, Quoc V. Le for his analysis recommendation and mentorship, and our senior writer Jeff Dean for his assist and deep technical discussions.
We additionally need to thank Ed Chi, Zoubin Ghahramani, Koray Kavukcuoglu, Dave Patterson, and Chris Manning for all of their recommendation and assist.