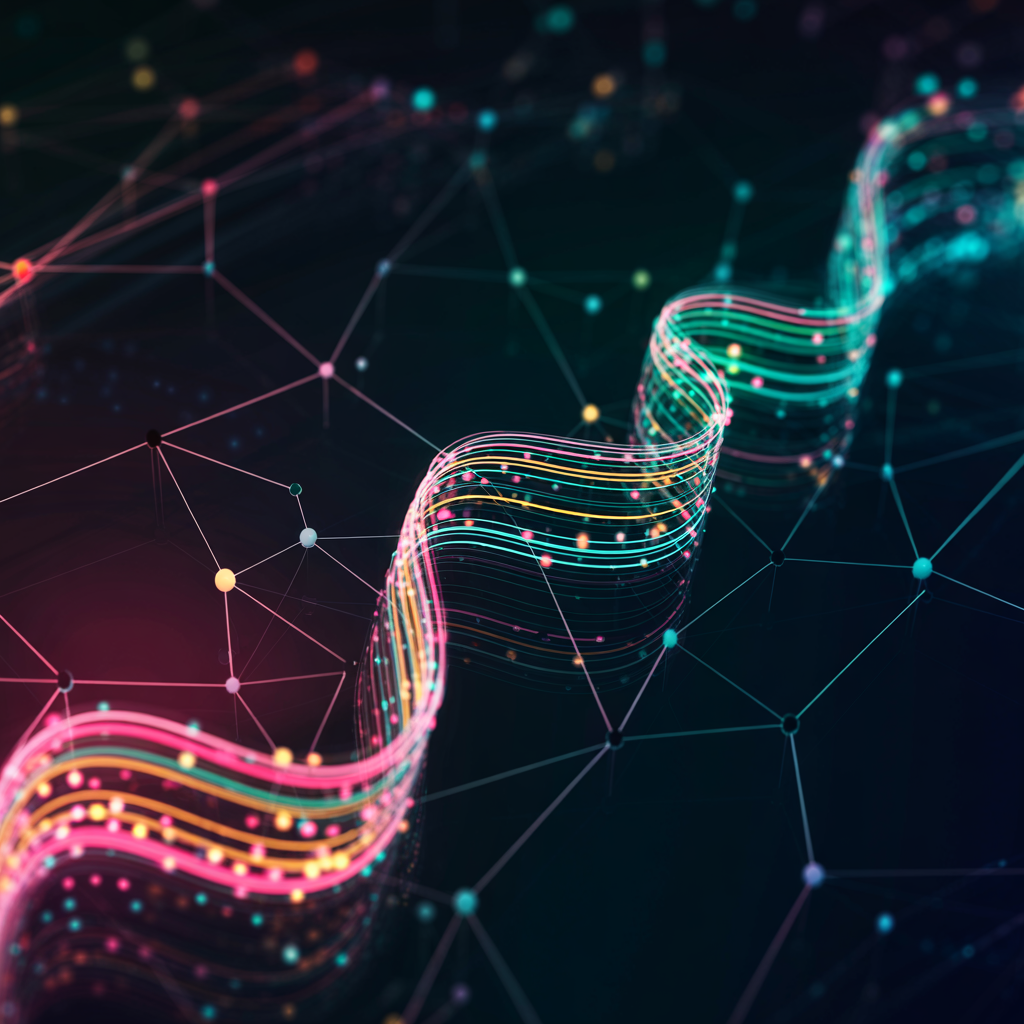
Deploying giant language mannequin (LLM)-based brokers in manufacturing settings usually reveals vital reliability points. Precisely figuring out the causes of agent failures and implementing proactive self-correction mechanisms is crucial. Current evaluation by Atla on the publicly accessible τ-Bench benchmark gives granular insights into agent failures, transferring past conventional mixture success metrics and highlighting Atla’s EvalToolbox strategy.
Standard analysis practices sometimes depend on mixture success charges, providing minimal actionable insights into precise efficiency reliability. These strategies necessitate handbook critiques of in depth logs to diagnose points—an impractical strategy as deployments scale. Relying solely on success charges, equivalent to 50%, gives inadequate readability concerning the character of the remaining unsuccessful interactions, complicating the troubleshooting course of.
To deal with these analysis gaps, Atla performed an in depth evaluation of τ-Bench—a benchmark particularly designed to look at tool-agent-user interactions. This evaluation systematically recognized and categorized agent workflow failures inside τ-retail, a subset specializing in retail customer support interactions.
Discover a preview of the Atla EvalToolbox (launching quickly) right here, and join to hitch Atla’s person group. If you want to be taught extra, e-book a name with the Atla crew.
An in depth analysis of τ-retail highlighted key failure classes:
- Workflow Errors, predominantly characterised by “Mistaken Motion” eventualities, the place brokers didn’t execute obligatory duties.
- Person Interplay Errors, significantly the supply of “Mistaken Info,” emerged as essentially the most frequent failure sort.
- Instrument Errors, the place appropriate instruments had been utilized incorrectly on account of faulty parameters, constituted one other important failure mode.
A vital distinction from this benchmark is the categorization of errors into terminal failures (irrecoverable) and recoverable failures. Terminal failures considerably outnumber recoverable errors, illustrating the restrictions inherent in agent self-correction with out guided intervention.
Right here’s an instance the place an agent makes a “flawed data” failure:
To deal with these challenges, Atla built-in Selene, an analysis mannequin immediately embedded into agent workflows. Selene actively screens every interplay step, figuring out and correcting errors in real-time. Sensible demonstrations present marked enhancements when using Selene: brokers efficiently corrected preliminary errors promptly, enhancing general accuracy and person expertise.
Illustratively, in eventualities involving “Mistaken Info”:
- Brokers working with out Selene persistently didn’t recuperate from preliminary errors, leading to low person satisfaction.
- Selene-equipped brokers successfully recognized and rectified errors, considerably enhancing person satisfaction and accuracy of responses.
EvalToolbox thus transitions from handbook, retrospective error assessments towards automated, quick detection and correction. It accomplishes this by way of:
- Automated categorization and identification of widespread failure modes.
- Actual-time, actionable suggestions upon detecting errors.
- Dynamic self-correction facilitated by incorporating real-time suggestions immediately into agent workflows.
Future enhancements embody broader applicability throughout various agent capabilities equivalent to coding duties, specialised area implementations, and the institution of standardized evaluation-in-the-loop protocols.
Integrating analysis immediately inside agent workflows by way of τ-Bench evaluation and EvalToolbox represents a sensible, automated strategy to mitigating reliability points in LLM-based brokers.
Notice: Due to the ATLA AI crew for the thought management/ Sources for this text. ATLA AI crew has supported us for this content material/article.
Asif Razzaq is the CEO of Marktechpost Media Inc.. As a visionary entrepreneur and engineer, Asif is dedicated to harnessing the potential of Synthetic Intelligence for social good. His most up-to-date endeavor is the launch of an Synthetic Intelligence Media Platform, Marktechpost, which stands out for its in-depth protection of machine studying and deep studying information that’s each technically sound and simply comprehensible by a large viewers. The platform boasts of over 2 million month-to-month views, illustrating its reputation amongst audiences.