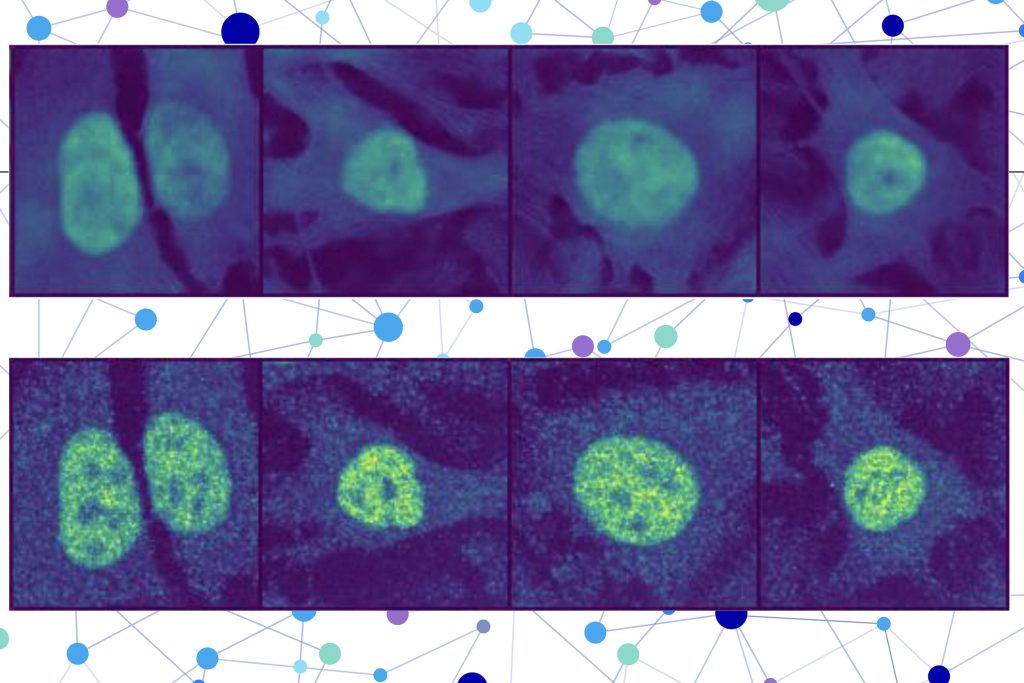
A protein positioned within the unsuitable a part of a cell can contribute to a number of ailments, corresponding to Alzheimer’s, cystic fibrosis, and most cancers. However there are about 70,000 completely different proteins and protein variants in a single human cell, and since scientists can sometimes solely check for a handful in a single experiment, this can be very pricey and time-consuming to establish proteins’ places manually.
A brand new technology of computational strategies seeks to streamline the method utilizing machine-learning fashions that usually leverage datasets containing hundreds of proteins and their places, measured throughout a number of cell traces. One of many largest such datasets is the Human Protein Atlas, which catalogs the subcellular conduct of over 13,000 proteins in additional than 40 cell traces. However as monumental as it’s, the Human Protein Atlas has solely explored about 0.25 p.c of all doable pairings of all proteins and cell traces inside the database.
Now, researchers from MIT, Harvard College, and the Broad Institute of MIT and Harvard have developed a brand new computational strategy that may effectively discover the remaining uncharted house. Their methodology can predict the placement of any protein in any human cell line, even when each protein and cell have by no means been examined earlier than.
Their method goes one step additional than many AI-based strategies by localizing a protein on the single-cell degree, reasonably than as an averaged estimate throughout all of the cells of a selected sort. This single-cell localization might pinpoint a protein’s location in a selected most cancers cell after remedy, as an example.
The researchers mixed a protein language mannequin with a particular sort of pc imaginative and prescient mannequin to seize wealthy particulars a few protein and cell. Ultimately, the consumer receives a picture of a cell with a highlighted portion indicating the mannequin’s prediction of the place the protein is positioned. Since a protein’s localization is indicative of its practical standing, this system might assist researchers and clinicians extra effectively diagnose ailments or establish drug targets, whereas additionally enabling biologists to raised perceive how advanced organic processes are associated to protein localization.
“You possibly can do these protein-localization experiments on a pc with out having to the touch any lab bench, hopefully saving your self months of effort. Whilst you would nonetheless have to confirm the prediction, this system might act like an preliminary screening of what to check for experimentally,” says Yitong Tseo, a graduate pupil in MIT’s Computational and Techniques Biology program and co-lead writer of a paper on this analysis.
Tseo is joined on the paper by co-lead writer Xinyi Zhang, a graduate pupil within the Division of Electrical Engineering and Laptop Science (EECS) and the Eric and Wendy Schmidt Middle on the Broad Institute; Yunhao Bai of the Broad Institute; and senior authors Fei Chen, an assistant professor at Harvard and a member of the Broad Institute, and Caroline Uhler, the Andrew and Erna Viterbi Professor of Engineering in EECS and the MIT Institute for Information, Techniques, and Society (IDSS), who can also be director of the Eric and Wendy Schmidt Middle and a researcher at MIT’s Laboratory for Data and Determination Techniques (LIDS). The analysis seems at present in Nature Strategies.
Collaborating fashions
Many present protein prediction fashions can solely make predictions based mostly on the protein and cell information on which they have been skilled or are unable to pinpoint a protein’s location inside a single cell.
To beat these limitations, the researchers created a two-part methodology for prediction of unseen proteins’ subcellular location, known as PUPS.
The primary half makes use of a protein sequence mannequin to seize the localization-determining properties of a protein and its 3D construction based mostly on the chain of amino acids that varieties it.
The second half incorporates a picture inpainting mannequin, which is designed to fill in lacking components of a picture. This pc imaginative and prescient mannequin appears to be like at three stained photos of a cell to assemble details about the state of that cell, corresponding to its sort, particular person options, and whether or not it’s beneath stress.
PUPS joins the representations created by every mannequin to foretell the place the protein is positioned inside a single cell, utilizing a picture decoder to output a highlighted picture that reveals the anticipated location.
“Completely different cells inside a cell line exhibit completely different traits, and our mannequin is ready to perceive that nuance,” Tseo says.
A consumer inputs the sequence of amino acids that kind the protein and three cell stain photos — one for the nucleus, one for the microtubules, and one for the endoplasmic reticulum. Then PUPS does the remaining.
A deeper understanding
The researchers employed a number of tips through the coaching course of to show PUPS tips on how to mix data from every mannequin in such a method that it might make an informed guess on the protein’s location, even when it hasn’t seen that protein earlier than.
For example, they assign the mannequin a secondary job throughout coaching: to explicitly identify the compartment of localization, just like the cell nucleus. That is accomplished alongside the first inpainting job to assist the mannequin be taught extra successfully.
A very good analogy is perhaps a instructor who asks their college students to attract all of the components of a flower along with writing their names. This further step was discovered to assist the mannequin enhance its normal understanding of the doable cell compartments.
As well as, the truth that PUPS is skilled on proteins and cell traces on the similar time helps it develop a deeper understanding of the place in a cell picture proteins are inclined to localize.
PUPS may even perceive, by itself, how completely different components of a protein’s sequence contribute individually to its general localization.
“Most different strategies often require you to have a stain of the protein first, so that you’ve already seen it in your coaching information. Our strategy is exclusive in that it might generalize throughout proteins and cell traces on the similar time,” Zhang says.
As a result of PUPS can generalize to unseen proteins, it might seize modifications in localization pushed by distinctive protein mutations that aren’t included within the Human Protein Atlas.
The researchers verified that PUPS might predict the subcellular location of latest proteins in unseen cell traces by conducting lab experiments and evaluating the outcomes. As well as, when in comparison with a baseline AI methodology, PUPS exhibited on common much less prediction error throughout the proteins they examined.
Sooner or later, the researchers need to improve PUPS so the mannequin can perceive protein-protein interactions and make localization predictions for a number of proteins inside a cell. In the long run, they need to allow PUPS to make predictions by way of residing human tissue, reasonably than cultured cells.
This analysis is funded by the Eric and Wendy Schmidt Middle on the Broad Institute, the Nationwide Institutes of Well being, the Nationwide Science Basis, the Burroughs Welcome Fund, the Searle Students Basis, the Harvard Stem Cell Institute, the Merkin Institute, the Workplace of Naval Analysis, and the Division of Vitality.