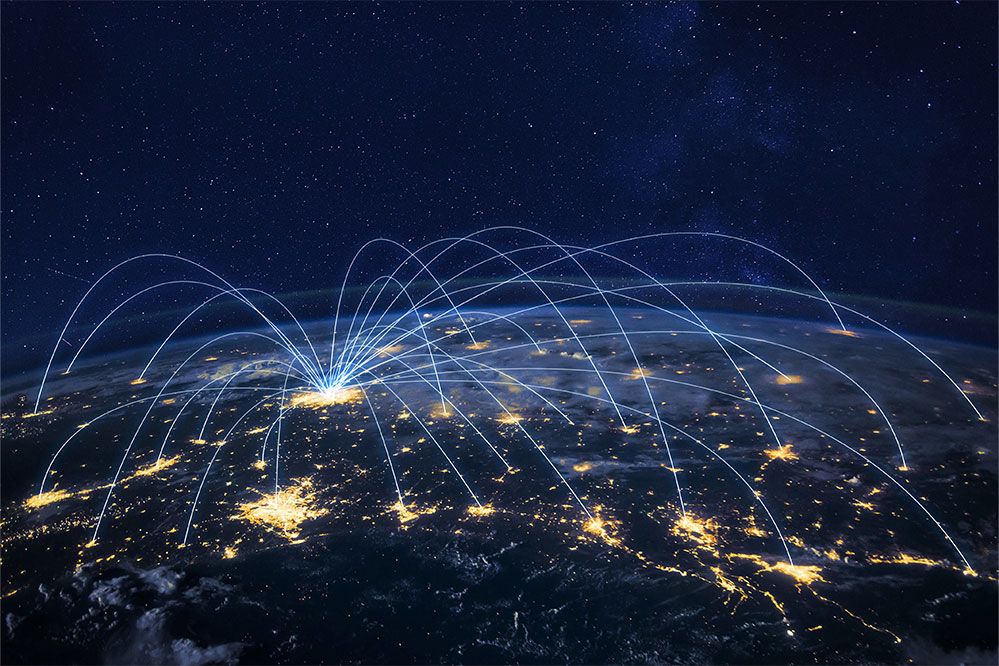
On Dec. 21, 2022, simply as peak vacation season journey was getting underway, Southwest Airways went by means of a cascading sequence of failures of their scheduling, initially triggered by extreme winter climate within the Denver space. However the issues unfold by means of their community, and over the course of the following 10 days the disaster ended up stranding over 2 million passengers and inflicting losses of $750 million for the airline.
How did a localized climate system find yourself triggering such a widespread failure? Researchers at MIT have examined this extensively reported failure for instance of circumstances the place methods that work easily more often than not all of a sudden break down and trigger a domino impact of failures. They’ve now developed a computational system for utilizing the mixture of sparse information a few uncommon failure occasion, together with far more intensive information on regular operations, to work backwards and attempt to pinpoint the basis causes of the failure, and hopefully be capable of discover methods to regulate the methods to stop such failures sooner or later.
The findings have been introduced on the Worldwide Convention on Studying Representations (ICLR), which was held in Singapore from April 24-28 by MIT doctoral pupil Charles Dawson, professor of aeronautics and astronautics Chuchu Fan, and colleagues from Harvard College and the College of Michigan.
“The motivation behind this work is that it’s actually irritating when we have now to work together with these sophisticated methods, the place it’s actually arduous to grasp what’s happening behind the scenes that’s creating these points or failures that we’re observing,” says Dawson.
The brand new work builds on earlier analysis from Fan’s lab, the place they checked out issues involving hypothetical failure prediction issues, she says, reminiscent of with teams of robots working collectively on a process, or advanced methods reminiscent of the ability grid, searching for methods to foretell how such methods might fail. “The objective of this challenge,” Fan says, “was actually to show that right into a diagnostic software that we may use on real-world methods.”
The thought was to supply a manner that somebody may “give us information from a time when this real-world system had a difficulty or a failure,” Dawson says, “and we will attempt to diagnose the basis causes, and supply slightly little bit of a glance backstage at this complexity.”
The intent is for the strategies they developed “to work for a reasonably common class of cyber-physical issues,” he says. These are issues by which “you’ve got an automatic decision-making part interacting with the messiness of the true world,” he explains. There can be found instruments for testing software program methods that function on their very own, however the complexity arises when that software program has to work together with bodily entities going about their actions in an actual bodily setting, whether or not it’s the scheduling of plane, the actions of autonomous autos, the interactions of a workforce of robots, or the management of the inputs and outputs on an electrical grid. In such methods, what usually occurs, he says, is that “the software program may decide that appears OK at first, however then it has all these domino, knock-on results that make issues messier and far more unsure.”
One key distinction, although, is that in methods like groups of robots, in contrast to the scheduling of airplanes, “we have now entry to a mannequin within the robotics world,” says Fan, who’s a principal investigator in MIT’s Laboratory for Info and Choice Techniques (LIDS). “We do have some good understanding of the physics behind the robotics, and we do have methods of making a mannequin” that represents their actions with affordable accuracy. However airline scheduling includes processes and methods which might be proprietary enterprise data, and so the researchers needed to discover methods to deduce what was behind the selections, utilizing solely the comparatively sparse publicly obtainable data, which primarily consisted of simply the precise arrival and departure instances of every aircraft.
“Now we have grabbed all this flight information, however there’s this whole system of the scheduling system behind it, and we don’t know the way the system is working,” Fan says. And the quantity of knowledge referring to the precise failure is simply a number of day’s value, in comparison with years of knowledge on regular flight operations.
The affect of the climate occasions in Denver in the course of the week of Southwest’s scheduling disaster clearly confirmed up within the flight information, simply from the longer-than-normal turnaround instances between touchdown and takeoff on the Denver airport. However the best way that affect cascaded although the system was much less apparent, and required extra evaluation. The important thing turned out to need to do with the idea of reserve plane.
Airways usually maintain some planes in reserve at varied airports, in order that if issues are discovered with one aircraft that’s scheduled for a flight, one other aircraft may be rapidly substituted. Southwest makes use of solely a single sort of aircraft, so they’re all interchangeable, making such substitutions simpler. However most airways function on a hub-and-spoke system, with a couple of designated hub airports the place most of these reserve plane could also be stored, whereas Southwest doesn’t use hubs, so their reserve planes are extra scattered all through their community. And the best way these planes have been deployed turned out to play a significant function within the unfolding disaster.
“The problem is that there’s no public information obtainable by way of the place the plane are stationed all through the Southwest community,” Dawson says. “What we’re capable of finding utilizing our methodology is, by trying on the public information on arrivals, departures, and delays, we will use our methodology to again out what the hidden parameters of these plane reserves may have been, to clarify the observations that we have been seeing.”
What they discovered was that the best way the reserves have been deployed was a “main indicator” of the issues that cascaded in a nationwide disaster. Some elements of the community that have been affected immediately by the climate have been capable of get better rapidly and get again on schedule. “However once we checked out different areas within the community, we noticed that these reserves have been simply not obtainable, and issues simply stored getting worse.”
For instance, the information confirmed that Denver’s reserves have been quickly dwindling due to the climate delays, however then “it additionally allowed us to hint this failure from Denver to Las Vegas,” he says. Whereas there was no extreme climate there, “our methodology was nonetheless exhibiting us a gentle decline within the variety of plane that have been capable of serve flights out of Las Vegas.”
He says that “what we discovered was that there have been these circulations of plane throughout the Southwest community, the place an plane may begin the day in California after which fly to Denver, after which finish the day in Las Vegas.” What occurred within the case of this storm was that the cycle acquired interrupted. Because of this, “this one storm in Denver breaks the cycle, and all of a sudden the reserves in Las Vegas, which isn’t affected by the climate, begin to deteriorate.”
In the long run, Southwest was compelled to take a drastic measure to resolve the issue: They needed to do a “arduous reset” of their complete system, canceling all flights and flying empty plane across the nation to rebalance their reserves.
Working with consultants in air transportation methods, the researchers developed a mannequin of how the scheduling system is meant to work. Then, “what our methodology does is, we’re primarily making an attempt to run the mannequin backwards.” Wanting on the noticed outcomes, the mannequin permits them to work again to see what sorts of preliminary situations may have produced these outcomes.
Whereas the information on the precise failures have been sparse, the intensive information on typical operations helped in educating the computational mannequin “what is possible, what is feasible, what’s the realm of bodily risk right here,” Dawson says. “That provides us the area information to then say, on this excessive occasion, given the area of what’s attainable, what’s the most definitely clarification” for the failure.
This might result in a real-time monitoring system, he says, the place information on regular operations are always in comparison with the present information, and figuring out what the pattern appears to be like like. “Are we trending towards regular, or are we trending towards excessive occasions?” Seeing indicators of impending points may enable for preemptive measures, reminiscent of redeploying reserve plane upfront to areas of anticipated issues.
Work on growing such methods is ongoing in her lab, Fan says. Within the meantime, they’ve produced an open-source software for analyzing failure methods, referred to as CalNF, which is out there for anybody to make use of. In the meantime Dawson, who earned his doctorate final yr, is working as a postdoc to use the strategies developed on this work to understanding failures in energy networks.
The analysis workforce additionally included Max Li from the College of Michigan and Van Tran from Harvard College. The work was supported by NASA, the Air Power Workplace of Scientific Analysis, and the MIT-DSTA program.