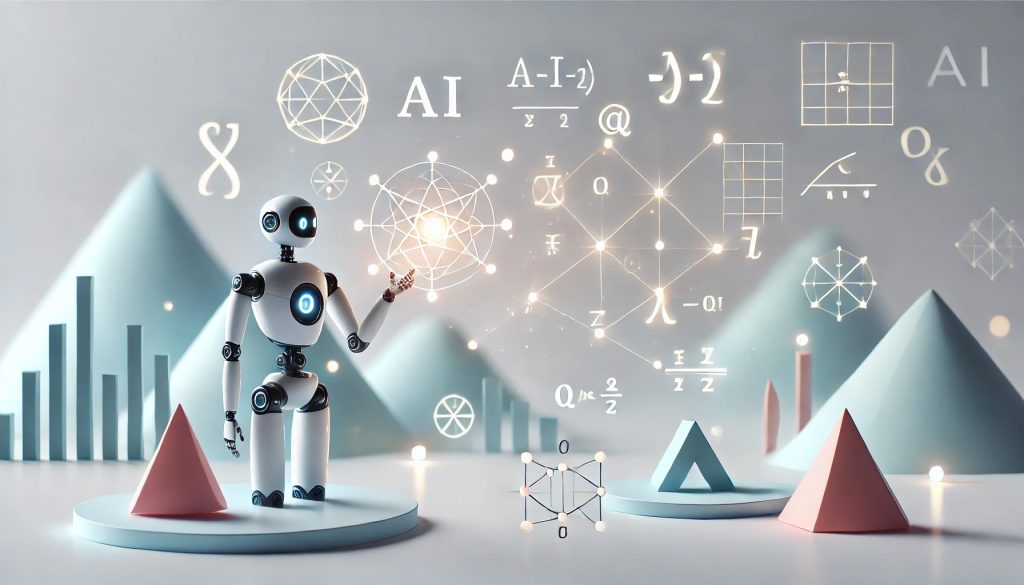
Unlocking AI Determination-Making with Arithmetic
Unlocking AI decision-making with arithmetic is remodeling the best way we perceive synthetic intelligence (AI). Think about a world the place machines are now not enigmatic forces working in a “black field,” however clear methods whose each resolution we will see, check, and belief. For innovators, researchers, and policymakers, this breakthrough isn’t nearly readability—it’s about advancing know-how whereas addressing its moral implications. Arithmetic has now emerged as the important thing to reaching this goal. For those who’re interested by how this revolution is going on and what it means for the way forward for know-how, you’re in the proper place.
Additionally Learn: Nineteenth-Century Automatons: Love and Warning
Why AI Determination-Making Is Usually a “Black Field”
The time period “black field” is usually used to explain AI methods as a result of their interior workings are usually opaque to people. The choices or predictions they make are primarily based on a posh mesh of interconnected algorithms and statistical fashions. Whereas these methods are extremely environment friendly at duties reminiscent of picture recognition, language processing, and information predictions, they not often clarify why they arrived at a selected conclusion.
This lack of transparency results in a number of challenges. For one, customers can’t determine whether or not an AI system is making truthful and unbiased selections. Secondly, when one thing goes flawed—reminiscent of incorrect medical diagnoses or discriminatory hiring selections—it’s virtually inconceivable to hint the foundation trigger with out understanding how the system works internally. These dangers have sparked a worldwide name for explainable AI options.
Additionally Learn: Exploring Click on-to-Do Recall on Home windows Copilot
The Promise of Arithmetic in Opening the Black Field
Arithmetic is enjoying an instrumental function in making AI methods extra clear and interpretable. By formalizing the interior mechanics of AI into mathematical fashions, researchers can translate the mysterious operations of algorithms into one thing understandable and reproducible.
As an illustration, breakthrough strategies like Shapley values—which come from cooperative recreation idea—are serving to to dissect machine studying fashions. This method assigns proportional “credit score” to every characteristic in a dataset for its contribution to a mannequin’s output. Such mathematical frameworks make sure that information scientists can quantify and contextualize how selections are made, whether or not it’s a monetary suggestion, a medical consequence, or a authorized evaluation.
Additionally Learn: Google Launches AI for Correct 15-Day Climate Forecasts
Key Challenges in Making Sense of AI Via Arithmetic
Whereas mathematical modeling provides nice promise, it’s not with out hurdles. One main problem is the sheer complexity of superior neural networks powering fashionable AI. These methods typically contain thousands and thousands—and even billions—of parameters, making them troublesome to distill into comprehensible formulation with out dropping accuracy.
One other issue arises from balancing transparency with efficiency. Some consultants argue that simplified mathematical fashions might sacrifice precision, which might result in defective or deceptive outcomes when utilized in real-world eventualities. Navigating this trade-off is a essential space of ongoing analysis.
A 3rd problem is person belief. Even when a mathematical rationalization is made accessible, will non-experts—reminiscent of medical doctors, judges, or shoppers—be capable to belief and successfully use the insights supplied? Addressing this challenge is critical for the widespread adoption of explainable AI options.
Additionally Learn: Anne Hathaway Leads Thrilling New AI Thriller
Explainable AI: A Rising Development
Explainable AI (XAI) has turn out to be a rising focus for researchers and organizations striving to bridge the hole between synthetic intelligence and human understanding. By making certain that AI methods can clarify their reasoning in an intelligible manner, XAI helps customers confirm and belief these methods.
A number of industries now demand XAI implementations to reinforce accountability. As an illustration, in healthcare, explainable fashions permit clinicians to know the premise of AI-generated diagnoses or therapy suggestions. Equally, in finance, regulators more and more push for transparency to stop bias or fraud in mortgage approvals and credit score scoring. The motion towards XAI is a transparent indication that transparency is now not non-obligatory—it’s important.
Using mathematical rules in AI isn’t only a technical development. It opens up a chance to deal with the profound moral questions surrounding know-how. By revealing how machines make selections, arithmetic helps stop misuse, cut back algorithmic bias, and promote equity.
A rising variety of researchers and builders are teaming up with ethicists to judge how AI impacts society. This multidisciplinary method ensures that AI methods will not be solely environment friendly but in addition aligned with human values and rules. Transparency performs a foundational function in fostering accountable AI innovation.
Examples of Sensible Purposes
The profitable use of arithmetic to decode AI is already making an influence. Take, as an illustration, the sphere of autonomous automobiles. Superior mathematical fashions are serving to researchers perceive why a automotive chooses one route, avoids one other, or reacts to sudden obstacles. Such insights are essential for making certain highway security and addressing legal responsibility considerations.
One other instance is seen in forensic AI methods. Legislation enforcement businesses more and more depend on AI options for prison investigations, however considerations over algorithmic bias in facial recognition and profiling abound. Mathematical transparency ensures these methods are scrutinized completely, boosting their accuracy and equity.
Even in artistic domains like artwork era and music composition, mathematical fashions are getting used to discover how AI makes aesthetic selections, bridging the hole between machine automation and human creativity.
Additionally Learn: AI Artist Sells $5M in Digital Artwork
Constructing Person Belief Via Clear AI
Understanding an AI system’s logic builds belief. When customers know why a system made a selected suggestion or resolution, they’re extra prone to interact with it confidently. Whether or not it’s sufferers trusting a prognosis instrument, workers counting on hiring software program, or shoppers interacting with digital assistants, transparency strengthens person reliance and satisfaction.
As belief grows, it additionally opens alternatives for broader adoption of AI. Many industries stay hesitant to totally implement these applied sciences as a consequence of fears of authorized legal responsibility or reputational hurt from opaque methods. Unlocking the decision-making course of eliminates these uncertainties, making AI safer for implementation worldwide.
Additionally Learn: ChatGPT Outage Sparks Hilarious Meme Craze
The Way forward for AI Transparency
As improvements persist, mathematical strategies for understanding AI will undoubtedly evolve. Researchers and builders are much more subtle methods, together with probabilistic fashions, causal inference strategies, and dynamic methods evaluation. These advances symbolize the subsequent frontier in making AI methods as clear as they’re clever.
Collaboration between academia, industries, and governments will likely be very important to speed up this progress. Requirements and rules might emerge to implement transparency necessities, making certain know-how builders prioritize explainability alongside efficiency metrics. The final word aim is to create methods that not solely excel of their duties but in addition acquire belief and acceptance from society.
Additionally Learn: AI Fixing Unsolvable Issues Past Human Comprehension
Why Unlocking AI Determination-Making Issues
The search to demystify AI decision-making with arithmetic is greater than a technical problem—it’s a societal crucial. Clear AI can enhance accountability, encourage innovation, and decrease hurt, making a basis for belief on this quickly advancing know-how.
Arithmetic, as a language of logical readability, is driving this transformation. By opening the “black field,” researchers and builders are setting the stage for an period the place AI methods will not be simply highly effective instruments but in addition trusted companions in fixing advanced challenges.
As you comply with this thrilling journey, keep in mind that understanding AI isn’t only for the tech-savvy. It’s a dialog concerning the type of future we need to construct—a future the place know-how serves humanity with readability, equity, and integrity.
Additionally Learn: Geoffrey Hinton Warns AI Might Trigger Extinction
References
Agrawal, Ajay, Joshua Gans, and Avi Goldfarb. Prediction Machines: The Easy Economics of Synthetic Intelligence. Harvard Enterprise Evaluate Press, 2018.
Siegel, Eric. Predictive Analytics: The Energy to Predict Who Will Click on, Purchase, Lie, or Die. Wiley, 2016.
Yao, Mariya, Adelyn Zhou, and Marlene Jia. Utilized Synthetic Intelligence: A Handbook for Enterprise Leaders. Topbots, 2018.
Murphy, Kevin P. Machine Studying: A Probabilistic Perspective.MIT Press, 2012.
Mitchell, Tom M. Machine Studying. McGraw-Hill, 1997.